Financial Services
Hyper-personalisation: The Next Frontier in Digital Transformation
26 February, 2024 | Written by: Krishnan Padmanabhan and Prashant Jajodia
Categorized: Financial Services
Share this post:
In recent years, the banking industry has witnessed a digital transformation, marked by a proliferation of features within mobile apps. However, the pursuit of adding more capabilities has reached a plateau, leading to a pivotal shift.
The era of the feature war is over. This evolution is no longer merely about incorporating additional functionalities; rather, it centres on tailoring the banking experience to individual needs and preferences making way for a new wave driven by hyper-personalised and proactive banking services, a move critical for enhancing customer satisfaction, engagement and most importantly customer loyalty.
To bring hyper-personalisation to life, let’s consider some examples.
- Proactive Nudges for Money management: Timely prompts to customers for reviewing subscriptions to services (some of which they may not need or have forgotten about), or for renewing a mortgage nearing the end of its fixed rate term or transferring money into savings shortly after receiving their salary credit. These help the customers with better visibility and control of their finances.
- Targeted Eco-system Play (Energy Bill): Harnessing data derived from smart meters, banks can take a proactive role in aiding users to optimize their energy expenses, providing insightful benchmark comparisons, usage tips and alternate deals, thereby contributing to an improved cost of living.
- Pre-approved offers for banking products: Based on their specific credit and behavioural history customers are presented with pre-approved loan offers for tailored amounts, surfaced as a banner upon login to mobile banking or internet banking.
- Improved customer service: AI models can glean insights from prior interactions and transactions to provide timely prompts and information to a customer agent while servicing a customer to provide a more personal service experience.
Challenges: The Roadblocks to Personalisation
While the concept of personalisation has been on the horizon for decades, the banking industry faces several challenges hindering widespread adoption:
- Regulatory Hurdles: Compliance with regulations like GDPR and navigating consent management poses obstacles to collecting and utilizing customer data.
- Data Quality and Fragmentation: Issues with data quality and fragmentation impede the seamless analysis required for effective personalisation.
- Organisational Silos: In larger institutions, the collaboration between data and digital teams is hindered by organizational silos, slowing down the implementation of personalised features.
- Technical Complexity: The technical framework for personalisation is non-trivial, demanding a combination of real-time and batch data analytics, complex rule systems, and continuous refinement based on customer interactions. Generative AI is a key enabler to unlocking this technical complexity.
The Building Blocks of a Personalisation Platform:
To deliver personalisation at pace, three building blocks have to be in place – Customer Data Platform, a Personalisation AI Brain, and Customer interaction framework.
A. Customer Data Platform: The foundation of personalisation lies in clean and comprehensive enterprise-wide data, with clear lineage. This consolidated data, often referred to as Customer 360, encompasses static customer master data, transaction data, interaction and complaints data (including audio transcripts), and external/social media data.
B. Personalisation AI Brain: In this area we see a multi-model solution emerging, with different use cases requiring different AI/analytics. Recent strides in Generative AI provides very powerful additional models for harnessing the information in more unstructured data.
The analytics use cases can be broadly classified into two types:
i. Batch Processes for Complex pattern recognition: Involves very large number of parameters and extensive historical data, demanding computational effort in pre-processing the “learning”. Examples include pre-approved loans where the output of batch analytics is the approved limit on the loan per customer, ready to serve upon the customer’s login.
ii. Real-time Analytics for Timely Alerts: Triggered by an event, providing timely alerts or nudges, but with less computational effort often requiring less “AI” but more deterministic logic. Examples include alerts like “you are one transaction away from your reward” or notifications about the receipt of a salary credit or even a potential fraudulent transaction.
C. The final foundational block is the framework for managing the way the output of any analytics is surfaced to the customer. This includes a push notification messaging system, an in-app clickable notification, or a clickable banner in the mobile app. Such a system dynamically handles the prioritization of messages, respecting customer preferences regarding the type of messages, channels, and timing. It also considers factors like the repetition of messages and ensures that any call-to-action hyperlinks to the right location for the action, complete with appropriate auto-population of data.
Approaches to Creating a Personalisation Platform:
The work IBM Consulting has been doing with various banks can be classified into three primary approaches to establish a robust personalisation platform:
- Implementing Comprehensive Products: Utilizing products like Personetics that provide a host of use cases out of the box. These products come with their own data models, analytic models, and a framework for pushing the output of personalisation to customer channels.
- Leveraging a comprehensive marketing or personalisation stack: Products such as Adobe, Customer.io, Bloomreach or Braze come with their own Customer Data Platform and Omni-channel orchestration, which can be integrated with custom-built analytics and rules.
- Build plus best-of-breed: Developing both the framework and specific use case analytics, often using products for some individual building blocks. This approach involves using a custom enterprise-specific data model on a data lake/warehouse plus a speed layer/operational data store (ODS), which is integrated with analytics engines like PEGA and customer messaging products Atomic.io to create a comprehensive personalisation platform.
This is still a nascent area for banks. In 2024, we will see some personalisation examples go live from neo-banks or high street banks who have in the past invested in a unified data platform, while others might be restricted to prioritising niche use cases where they have the data.
As banks navigate these building blocks and approaches, the promise of a more tailored, engaging, and proactive banking experience awaits their customers.
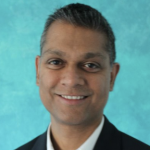
Partner, IBM Consulting
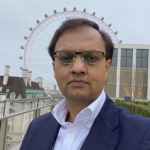
Financial Services Sector Leader and Managing Client Partner, IBM Consulting UKI
Converting website traffic into happy customers with a smart virtual assistant
With a long track record of guiding companies across various sectors through digital transformation, IBM Business Partner WM Promus is now focusing AI innovation. Eileen O’Mahony, General Manager at WM Promus, explains how her company helped a UK-based commercial finance brokerage enhance customer experience, and develop new sales leads using IBM watsonx and IBM […]
Reducing the time taken to write regulatory submissions – Introducing our Accelerator
The Case for Generative AI in Regulatory Acceleration Generative AI and automation are now enabling digital transformation across biopharma, allowing radical reshaping and automation of core processes – and focusing human effort where it is required. Companies embracing this approach across the whole organisation are deriving significant competitive advantage and transforming the way work is […]
Impact on Data Governance with generative AI – Part Two
Many thanks to, Dr. Roushanak Rahmat, Hywel Evans, Joe Douglas, Dr. Nicole Mather and Russ Latham for their review feedback and contributions in this paper. This blog is a continuation of the earlier one describing Data Governance and how it operates today in many businesses. In this blog, we will see how Data Governance will […]