Data & AI
The rise of the data scientist
12/11/2014 | Written by: Think Blog redactie (0cB)
Categorized: Data & AI
Share this post:
Written by: Marc Teerlink, Global Strategist & Chief Data Scientist at IBM and
Olav Laudy, Worldwide Predictive Analytics Solutions Leader at IBM
In the film Moneyball, Billy Beane takes over as manager of the Oakland Athletics baseball team in California. With the club in dire financial straits, he adopts a highly unorthodox strategy for achieving results.
To scout for possible new players and build up a team to compete in the World Series, he uses a computergenerated analysis. During the 2012 US presidential elections, Barack Obama’s campaign was run as the political equivalent of Moneyball: A numerical analysis preceded every decision made by the campaign, with the analyses conducted by a small team headed by data scientist Harper Reed. Another example is Nate Silver, an American statistician who gained fame after accurately predicting the outcomes of the 2008 and 2012 presidential elections. Silver launched his data blog FiveThirtyEight (www.fivethirtyeight.com) in 2008. With experience in Moneyball-like sports statistics, Silver accurately forecasted the election results for 49 of the 50 states and for 35 of the senate races in 2008 – all by making algorithm-based predictions on the data known as Big Data.
What is Big Data?
At the most basic level, what makes Big Data “big” is simply that there is a lot of it. The size and speed with which all this data is now being generated means it can no longer be processed using traditional technology. But Big Data is not just about its size; it is also tied to issues of variable speed, veracity and variation. Data is found in limitless forms and formats, from the brand of toothpaste you bought yesterday to your precise location when you phoned the office. There is also greater variation in data types than are in all those commonly cited lists, formulas and databases – such as data sets with different structures collected from sensors, books and documents, images, sound and GPS locations; or those obtained by physical measurements you take yourself. Data scientist: the sexiest job When the Harvard Business Review announced in 2012 that data scientist was the “sexiest job of the 21st century,” the Twittersphere exploded with the cheers of econometrists, operations research professionals, actuaries and statisticians.
But most tweeters weren’t even sure what data scientists did. That is set to change rapidly in the next few years, as an increasing number of companies seek to reap the benefits of Big Data and the role becomes more defined. Previous research has already demonstrated that data analysts play an essential role in successful companies, and it is expected that that will only grow. But what is now also becoming clear is that when decisions are being made the message emerging from the data is not always usable. Sharing data is the new form of having data Business analysts are frequently incapable of providing what the decision-making process needs most – insight; not just the figures. And so data scientists must have a sense of curiosity, must closely scrutinize data for a lengthy period, and must pick up on trends. The data scientist must be interested in everything – not just the business aspects – and must want to change an organization. He must be the link between business and data: What does Big Data tell us about our processes and how can we perform better and faster? The data scientist has to do more than merely analyze data or create models, and unlocking data is just a small part of the job description. He also has to visualize that data so that the analysis can become more comprehensible to everyone else, and as a result be used predictively rather than reactively.
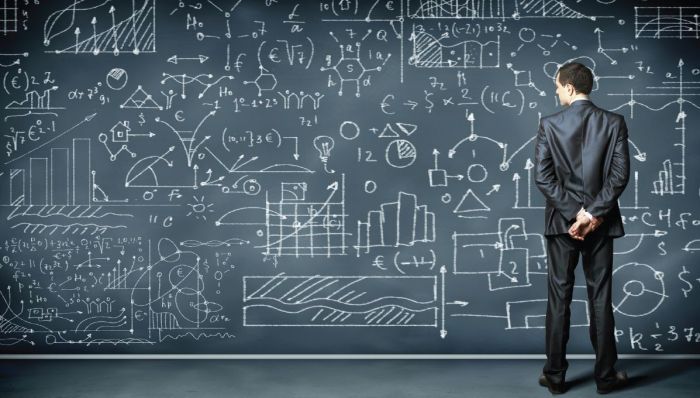
Data science, where to start, three tips!
Tips for the starting data scientist
1. Start with the question, not with the Big Data,
and answer the right question hen using Big Data, it can be tempting to hunt out the answers to a wide variety of interesting questions. But, after all the analyses are conducted, if the focus has been on questions nobody in the company asked, you’ll be adding unnecessary extra elements to the story at the expense of finding the answers that are critically important to decision-makers. Fundamentally, therefore, your primary task as a data scientist is to help people formulate better questions.
2. Tell the story, speak your company’s language
Data scientists like their data. But if you want your voice to be heard, you will have to translate your findings into the language that your intended audience speaks. Start off with the answer to the “So what?” question instead of launching into an explanation of the methodology. Use dynamic visualizations to bring Big Data to life.
3. Be modest, and validate the results with others
Most data manipulators sometimes fall into the trap of believing that their data ref lects the total reality of the situation. We wrestle our way through the data and arrive at a solid, fascinating and controversial conclusion. Our instinct is then to approach the nearest decision-maker and say, “Look at this! You have to change the company!” But it is quite possible that we do not have the whole picture, and a few cry wolfs can easily undermine the credibility of Big Data Analytics. So it is essential that you first validate a number of assumptions and conclusions with people of experience who understand the company well. Give them the chance to come on board and share the credit. And listen to them, for it can only be a win-win situation.
Conclusion
Thanks to the technology currently available, collecting and unlocking Big Data has become simple. But it is more difficult to truly understand that data, and the real reward only comes when you can effectively communicate those insights. As Silver says, the more humility we have about our ability to make predictions, the more successful we can be in planning for the future.
Data-driven asset management with IBM Maximo Application Suite and Cloud Pak for Data
IBM has enhanced its Enterprise Asset Management platform, IBM Maximo Application Suite (MAS), with IBM Cloud Pak for Data: a supporting platform which provides a framework for combining a variety of data from different areas of an organization. How does IBM Cloud Pak for Data help organizations gain additional asset management insights from available data? […]
Being a data driven organization: What does this mean at Allianz?
Reading Time: 8 minutes From calculating risks and premiums to understanding customer behavior, data is of vital importance in the insurance business. Allianz, a multinational financial services company that focuses on insurance and asset management, has recently transformed its operations on a data level to reinvent its insurance business. Read an extract from a recent […]
How data analytics and AI push the frontiers of research for high-impact innovation at LIST
Data analytics and AI are more than just buzzwords when it comes to research and innovation. From handling big data to uncovering new insights, data analytics and AI have proven their value time and time again at LIST. Introducing LIST LIST, also known as the Luxembourg Institute of Science and Technology, is a […]