AI
Putting Diversity to Work in Data Science
December 19, 2019 | Written by: Seth Dobrin, PhD and Susara van den Heever, PhD
Categorized: AI | Data Science | Diversity & Inclusion
Share this post:
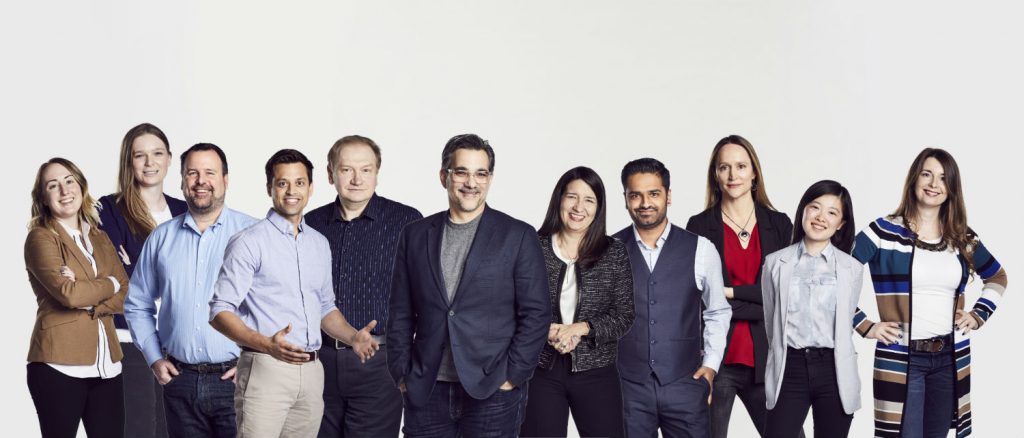
Seth Dobrin, (center), Susara van den Heever (third from right), and members of the IBM Data Science Elite Team.
Gender diversity of data scientists has been cited as an issue by many sources. According to The Burch Works Study of Data Scientists, only 15% of data scientists are female and the percentage of females in data scientist manager roles (<10%) is much less than that of early professionals (> 20%). This data is consistent with studies from other organizations. Although such statistics may lead one to believe that a skills gap is wholly to blame, we recognize there is more at play.
There is ample evidence that shows that women are graduating in vast numbers with scientific and technical degrees. In 2012, 42% of European PhDs in science, maths, and computing, were awarded to women. In the U.S. in 2019, women comprised 43% of the workforce of scientists and engineers. Additionally, there are female focused data science groups that have tens of thousands of participants. For example, the Global Women in Data Science (WiDS) Conference, attracts more than 100,000 participants each year.
Against this backdrop, the question then becomes, how can we fix this?
The Business Value of Diversity
Let’s begin with another stat. In addition to fairness, diversity drives business value. A recent McKinsey report shows that “companies in the top quartile for racial and ethnic diversity are 35 percent more likely to have financial returns above their respective national industry medians,” and that, “companies in the top quartile for gender diversity are 15 percent more likely to have financial returns above their respective national industry medians.”
Diverse teams are also more likely to make fact-based decisions with more accurate group-thinking, and are more innovative, as reported in the Harvard Business Review. There is additional evidence that diverse teams make companies more productive include a Harvard Business Review Study from 2019.
How to Attain It
To fix the diversity problem each and every one of us has to look deep inside ourselves and fix our own biases, and then put in place concrete action plans to find and hire diverse talent. These plans must encompass the entire talent acquisition pipeline.
But first a personal story from Seth: “In 2013 I set out to hire a very, very scarce subset of data scientist. Not only was I having trouble finding qualified candidates but there was zero diversity in the candidate pool. I was speaking with my wife about it and she brought up a good point that my job description was essentially a wish list: I want someone with these 25 ‘things.’ She said the things on the list were not all skills that were necessarily required for success. We worked together to convert the description into a menu: 3 of these, 2 of those, 1 of these. The results, while anecdotal, were immediate. The pool of qualified candidates doubled entirely by adding women, people of color and other underrepresented groups. In the end I hired a very qualified candidate who also happened to be Latino, complimenting my team with both new skills and racial diversity. This helped set my frame of mind in my next mission and fundamentally changed my approach to hiring moving forward.”
Applying Diversity to the IBM Data Science Elite Team
Beginning in early 2018 we set out to create the IBM Data Science Elite Team with a goal of hiring about 100 data scientists. This group would be a client-facing organization dedicated to walking customers through assessment and planning of data science projects as they mapped out their individual roads to AI. Hiring was to be done in 3 sprints of 3 months, each spread over the course of a year. An additional goal we set for the leaders of this team, was to hire a highly skilled and diverse team. Ultimately, we wanted the team to be comprised of the most qualified candidates, including women and other diverse candidates.
Our approach was essentially to reevaluate the entire hiring cycle, from writing the job descriptions to making the candidate an offer. Here’s how we outlined the approach:
- Job Descriptions: Our initial step was to create job postings and descriptions deliberately designed to not discourage underrepresented groups from applying for the roles. An HBR article by Tara Sophia Mohr demonstrated that men are twice as likely to apply to a job posting that they are not 100% qualified for. This is largely due to the perception of women that hiring requirements are, in fact, required. Men, on the other hand, are more likely to view a list of requirements as a menu of items to select from in order to showcase the skills that would serve them well in the role. Based on this study and past experiences we crafted job descriptions that read as more variable skills and used gender-neutral words.
- Final Candidate Pool: The next thing we did was to establish a unique new rule: no one could begin the interviewing process until the pool of qualified candidates was diverse. The diversity of specific pools was inferred and was only checked with actual statistics at the macro level. It’s important to note that we had no requirements on the demographic of who would be hired from this pool. This approach is supported by evidence from another HBR study, one by Stefanie K. Johnson, David R. Hekman and Elsa T. Chan in 2016. The results of their study found that if there is only one woman or other underrepresented minority in a candidate pool that person essentially had zero chance of getting hired. Additionally, they found that when having at least two women or two minorities in a hiring pool the odds of hiring a woman or person of color were 79.14 times greater and 193.72 times greater respectively. This is regardless of the overall size of the candidate pool.
- Interview Process: We created a highly-rigorous interview process consisting of shallow technical interviews, deep technical interviews, coding challenges, and soft-skill interviews. This process was carried out by a diverse hiring team. We had skills standards and we stuck to them.
Diversity Works
By following this process, we have built a global team with diversity that is much higher than is seen on the market. This team is highly skilled and has worked closely in small groups with more than 100 different IBM clients. Our anecdotal evidence of the team’s exceptional work typically comes in the form of personal exclamations, such as, ‘How did you put this team together? They are awesome!’ Not surprisingly, such accolades are generally followed by comments about the level of diversity. One client expressed their joy in seeing that our diversity-focused approach is breaking the age-old corporate culture of “I know a guy” – we are hiring and advancing people because of their talent, not because of who they might know.
A personal story from Susara, who led the hiring of the European IBM Data Science Elite team: “Seth gave me the mission to build a diverse top-talent Data and AI team from the ground up. I was fortunate to work with a boss and mentor who provided training to recognize and address bias, who put in place a diversity-focused hiring practice, and who motivated me and my peers to build a diverse top talent team. Today my team of 25 consists of 43% women, we have 18 different nationalities, and we speak 25 different languages!”
While we believe we can be more diverse, we are very close. Worldwide, we have female representation that is about 75% higher than the norm. We are roughly 20% PhD’s, 60% Masters’ and 20% Bachelors’ graduates. We are also proud of the racial and LGBTQ diversity we have been able to build within our teams but also recognize that the work of diversity and inclusion is perpetual for teams who strive to do it well.
Our goal was to create a diverse team of highly technical and highly talented individuals, not only because it’s the right thing to do, but because it drives better business outcomes. There’s more to be done, and we’re constantly working on improvements. But we’re thrilled with the results thus far.

Vice President, IBM Data and AI and CDO IBM Cloud Computing and Cognitive Software

Executive Data Scientist and Program Director, IBM Data Science & AI Elite
Making the workplace safe for employees living with HIV
The recent promising news about Covid-19 vaccines is in sharp contrast to the absence of a vaccine for HIV, despite decades of research. Unlike Covid-19 with a single viral isolate that shows minimal diversity, HIV circulates in a wide range of strains that so far have proven impervious to a single vaccine. Fortunately, more people […]
Call for Code for Racial Justice Needs You: Join the Movement
IBM has never avoided taking on big challenges. At IBM, we are privileged to drive impact at scale. We take on challenges that transform our clients, impact people’s lives and innovate for future generations as we strive to effect systematic societal change. Over the course of our 109-year history, the evidence has become clear that […]
A New Wave: Transforming Our Understanding of Ocean Health
Humans have been plying the seas throughout history. But it wasn’t until the late 19th century that we began to truly study the ocean itself. An expedition in 1872 to 1876, by the Challenger, a converted Royal Navy gunship, traveled nearly 70,000 nautical miles and catalogued over 4,000 previously unknown species, building the foundations for modern […]