This Is Watson
The 3 Beachheads of AI
October 16, 2019 | Written by: Rob Thomas
Categorized: Data Analytics | Data Science | Hybrid Multicloud | IBM Watson | Machine Learning | This Is Watson
Share this post:
(Part 2 in a Series) We have been partnering with and assisting clients on their data needs and strategies for years. It’s clear that data and AI are two-sides of the same coin; in fact, this understanding spawned the AI Ladder concept. We’ve developed skills training in the areas of data science and machine learning; we’ve partnered with standards bodies to create new certifications around data science; and we even created a group of experts, the IBM Data Science Elite Team, which is dedicated to helping clients get this first AI model into production, on our nickel.
Based on our experience, I have identified three areas (or beachheads) that are essential to leading in AI: a Differentiated Platform, Skills and Research. Let’s decompose each one.
A Differentiated Platform
It is important to note that more than 90% of AI technology is “common knowledge.” So, what matters is where a company puts its focus in technology hardening and productization. IBM Watson, out of necessity (lack of consumer data, extreme caution on using public datasets, AI customization required by enterprise customers, etc.), has focused on productizing technologies that will let customers train/customize models with less data and expertise.
In contrast, you see companies like Google and Amazon who hire thousands of contractors to improve base models and do data labeling to improve their base models for every conceivable situation. That is not the business we are in. There are a few things that I think make us unique:
- Ease of AI customization. For example, intent classification (the heart of Watson Assistant) allows organizations to learn from small training sets. This means you don’t need to hire an army of data labelers. This technology is driving huge market successes like RBS (mentioned above), Bradesco, Credit Mutuel, Vodafone UK, and more.
- Automation of AI. For example, our AutoAI technology gives the average data scientist superpowers. We automate 80% of core data science processes like algorithm selection, feature engineering, and data preparation. This is a unique capability of Watson Studio (a place to build/train models).
- Explainability of AI models. In a world where trust matters, every organization will want the comfort (and compliance) of knowing how their AI is making decisions. Our software enables users to understand how their models are performing, drift, where bias may exist, and how to explain the answers. And, this works across any AI tools, not just the ones from Watson.
- Embedded capabilities. Any company can pick up Watson and make their own products better.
Are we perfect? No, of course not. Is this great software? Yes. But you don’t have to believe anything I say – the tools are easy to try yourself.
Skills
The biggest challenge organizations are facing is depth and breadth of skills to apply data science and AI. Companies get excited about AI, but when it comes to scaling AI in their organization, their data scientists are too few and far between. There are two ways to attack the skills deficit:
- Automation. Any time there is a mismatch between supply (skilled workers) and demand (for a particular skill), automation comes to the forefront. With things like the aforementioned AutoAI, we believe that we are making average data science skills superb. We also help train people in the first place, with things like the AI Skills Academy that we launched in February.
- Expertise. Last year, we built a team called Data Science Elite. These are some of the world’s best data scientists, that we put onsite with clients at no charge. Their mission is to build, train, and get an initial model into product. A single success will galvanize an entire organization.
And many successes we have seen. The client endorsements speak for themselves, as you can see here:
“Working with the Data Science Elite team was an important accelerator for us,” said Adam Woods, CTO at Wunderman Thompson. “We were committed to moving toward a fully distributed architecture for our machine learning but one of the biggest challenges we faced was resources. Our teams having the ability to free up time from their day-to-day responsibilities to learn and build a proof of concept organically. With the Data Science Elite team, we were able to collaborate closely. Our data scientists focused on the immediate business requirements and the IBM team focused on the technology. This joint collaboration resulted in a machine learning pipeline, via Watson, that fully utilized all of our data signals to produce models that increase the performance over our previous models by 200% or more. We are now working aggressively to roll this out into our production.”
Research
To stay relevant in AI, we must be finding and leading the new frontiers. IBM has seen 93% YTY growth in research conference papers published. There is an increasing impact of our investment in the MIT-IBM Watson AI Lab, as represented by citations. And, IBM is in the top 10 of organizations with patents in supervised and unsupervised learning. We remain one of the few companies with a research organization dedicated to the topic of innovation in AI, and it is something in which we take much pride.
Earlier this year, we unveiled Project Debater, at IBM’s THINK 2019 Conference. This is an AI demonstration, that shows a system participating in a live debate with expert human debaters. In fact, it’s the first and only AI-powered computational argumentation tool, that can absorb information and build persuasive arguments. Natural Language Processing (NLP) is at the heart of Debater, which is one of the reasons I refer to NLP as the nervous system of AI.
_______________________________
NOTE: In the spirit of predictive analytics, in Part 3 of my series due next week, I will share what I believe the future AI will look like.
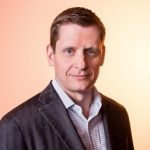
Senior Vice President, IBM Cloud and Data Platform
Accelerating Digital Transformation with DataOps
Across an array of use cases, AI pioneers are employing a core set of new AI capabilities to unlock the value of data in new ways. According to the 2019 IBM Global C-suite study, leaders are using data 154% more to identify unmet customer needs, enter new markets, and develop new business models. These leaders […]
IBM Watson Makes the GRAMMYs Pop
I am a child of the ’80s, raised on a steady diet of fruit rollups and music videos. My most enduring memories of high school are set at my girlfriend’s house, sitting in her den, watching the videos that became the soundtrack of our relationship. At the time, I was convinced that music videos were […]
AI in 2020: From Experimentation to Adoption
AI has captured the imagination and attention of people globally. But in the business world, the rate of adoption of artificial intelligence has lagged behind the level of interest through 2019. Even though we hear that most business leaders believe AI provides a competitive advantage, up until recently, some industry watchers have pegged enterprise adoption […]