Data Science
New Roche Study: Predicting Diabetes-Related Chronic Kidney Disease with Real-World Data
March 1, 2019 | Written by: Lars Boehm
Categorized: Data Analytics | Data Science
Share this post:
Chronic kidney disease (CKD) is one of the most severe secondary complications related to diabetes. It is characterized by the progressive loss of the kidney function, beginning with a decline in the glomerular filtration rate and/or albuminuria, eventually resulting in end-stage renal disease. This severe complication often requires dialysis or renal transplant therapy.
In January 2019, we released a study, showing the impact of predictive analytics based on real-world data. Our work, published in Nature Medicine, has shown that a newly developed predictive model based on real-word data appeared significantly more accurate in early risk identification to develop CKD than traditional methods. As a result, this is a promising step towards improved, personalized healthcare aiding clinical decision making.
In this blog I share further insights on how we achieved these substantial results and what they mean for patients and the industry.
Understanding the needs
At the beginning of this work, I had the privilege to get in touch with people living with diabetes. This remarkable experience gave me an initial idea about the challenges to manage a life with this chronic disease. Next to the constant hassle to manage blood sugar levels 24 hours a day, the anxiety of long-term consequences was a prominent theme in the conversations.
The risk of long-term consequences such as retinopathy, cardiovascular complications, kidney failure, etc. is elevated for any person living with diabetes. We asked ourselves: “Could early warnings be more specific to identify and support individuals at risk?” It was obvious that there is still much potential to provide relief for people with diabetes.
Defining the key objective and mapping the foundation
With advancements in AI and the accessibility of data in biomedicine there are huge opportunities to improve outcomes in diabetes. We adopted an approach driven by user needs and created a master list of potential initiatives mapped against data-related opportunities. The need related anxiety about long-term complications stood out and we set our key objective: Advancing diabetes care in the area of long-term complications by leveraging real-world data.
But there was no time to launch a long-term clinical study to analyze diabetes-related complications. At the same time the plethora of real-world medical data in clinics and medical doctors’ offices provided great potential to help evaluate patient’s risk level and tailor the most appropriate treatment. The availability of such data for secondary research within the IBM Explorys helped to sidestep a clinical study.
In order to achieve outstanding results two factors are critical for success: Agility and a multidisciplinary approach. In our method, we emphasize the study questions that are related to the key objective and focus on generating tangible results quickly.
Agility in real-world data science
In areas where huge data sets are involved and ground-breaking innovation is the ambition, it is important to retain agility along the way. There are multiple influential factors which are usually discovered throughout the project and which require the research team to flexibly adapt. First, real-world data itself holds surprises requiring flexibility to adjust inclusion and exclusion criteria, outliers, data features. Second, the modelling technique needs to align data-driven and medical insights. Third, there needs to be flexibility to allow for validation based on additional independent real-world data sets.
To retain agility our approach follows a couple of principles:
- A constantly prioritized backlog of broken-down study questions.
- An overall plan that consists of multiple analysis iterations.
- Creation of tangible insights that are medically and scientifically benchmarked.
A multidisciplinary approach
This work was a truly collaborative effort across organizations building on the strength of each participating party and following nine instrumental disciplines which need to be mastered as a team: 1) medical expertise in the disease area, 2) excellence in data science 3) strong programming in data related technologies 4) visionary thinking 5) health economic understanding 6) scientific approach 7) stakeholder engagement 8) agile project management 9) expertise in electronic patient data
Achieving impact
Adopting the described practices, the team has created an algorithm for practical use that outperforms traditional approaches. The supervised selection of a limited number of features allows the practical use of the algorithm in various scenarios, including potential integrations into digital health systems.
This is a promising first step since these new predictive models may provide an efficient tool for improved, personalized healthcare aiding clinical decision making. They offer the potential for a timely mitigation of the impact and severity of diabetes-related complications. The approach and methods described in this blog can even generate an impact in other therapeutic areas beyond diabetes.
____________________________________________
Further Information:
- The New Roche Study: Press Release
- Nature Medicine: Predicting the early risk of chronic kidney disease…
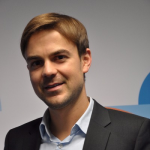
IBM Associate Partner, GBS Watson Health Consulting Leader, CH
Accelerating Digital Transformation with DataOps
Across an array of use cases, AI pioneers are employing a core set of new AI capabilities to unlock the value of data in new ways. According to the 2019 IBM Global C-suite study, leaders are using data 154% more to identify unmet customer needs, enter new markets, and develop new business models. These leaders […]
Medicine and the Message: Consumer Engagement and the Power of Nontraditional Data
The journal Health Affairs estimates that the U.S. spends nearly $500 billion annually on pharmaceutical treatments. Focusing on clinical value alone isn’t enough to meet business growth targets — customer experience plays a critical role in long-term brand success. But experience doesn’t occur in isolation: To win trust and boost spending, pharma brands must use […]
University of Oklahoma Taps AI to Strengthen Student Retention Rates
Graduating from a four-year college in four years should be an achievable goal, but only just over 40 percent of students are able to reach this milestone. A critical driver for achieving on-time graduation is first-year retention. But for public institutions, like the University of Oklahoma (OU), the national average of full-time, first-time students who started […]